From data to decisions: Exploring the power of the AI dashboard
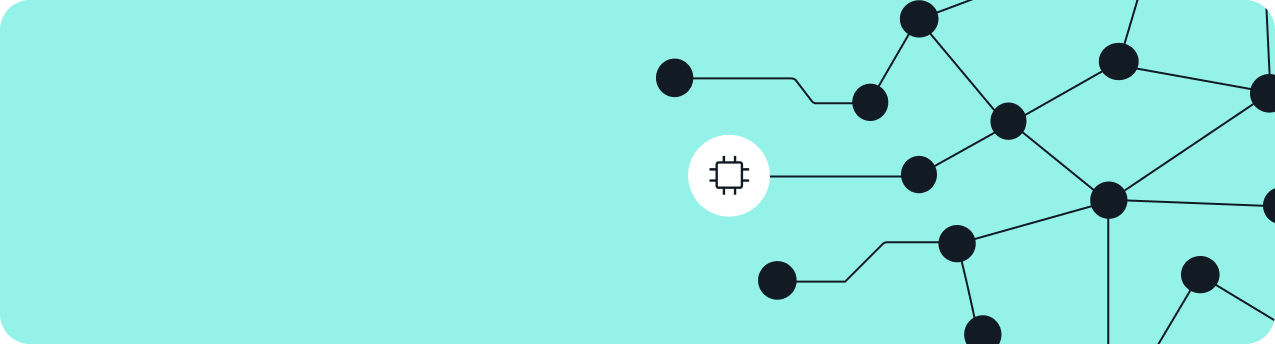
- Blog
- AI/ML
Discover the transformative potential of AI dashboards in unlocking advanced analytics and informed decision-making across industries.
Dashboards have become essential for business users to monitor performance and gain insights. However, most traditional dashboards are limited to descriptive analytics, providing a rear-view mirror summary of historical data and past events. It’s been mostly unchanged for decades.
With the accelerating advancements in AI, end-users are now demanding more. A Harvard Business Review article “GenAI Is Making Companies More Data Oriented,” shared a survey reporting that the percentage saying their organizations had “established a data and analytics culture” increased from 21% to 43% year-over-year and that organizations who had “created a data-driven organization” doubled from the prior year—the biggest uptick in the history of the survey. McKinsey noted that companies growing their investments and interest in AI and all things data science, driven by GenAI buzz, reported that 40 percent of respondents say their organizations will increase their investment in AI.
With all of this, end-users are more aware of what’s possible than ever, and they now expect more than plain old descriptive analytics in embedded in the apps they use. Advancements in data science have paved the way for a new kind of dashboard—an AI dashboard.
An AI dashboard goes beyond descriptive analytics and incorporates predictive analytics, prescriptive analytics, and natural language copilot experiences. Descriptive analytics focuses on summarizing historical data and providing insights into past events. For product managers, the goal is to empower users with the ease of use and consumption they are accustomed to in a dashboard experience, all while enabling end-users with more powerful decision-making by infusing data science. In fact, a recent Gartner survey on data and analytics practices reported, “Self-service analytics is critical to scaling the benefits of data-driven decision-making.”
In this article, we’ll discuss valuable use cases for AI dashboards across industries: sales and marketing, finance and accounting, and retail. We’ll also share some critical considerations for the design and development of AI dashboards, which apply to all industries.
How are today’s AI dashboards different from yesterday’s?
If you or your end-users are using a dashboard today, chances are it’s pre-AI. Most current dashboards are typically almost entirely descriptive. For example, a dashboard geared around descriptive analytics might show visualizations and metrics that include year-over-year pricing changes, month-over-month sales growth, or how revenue per customer has been tracking for the last few periods.
Dashboards built to deliver descriptive analytics have been around for decades, but they remain a powerful way for users to compare performance and trends within a business, identify patterns and trends, and gain key insights.
But now, data science has broken out of the backroom and is shifting to the frontlines of decision-making. The opportunity is to provide a dashboard with AI-based insights infused into it—an AI dashboard. A key design goal of an AI dashboard is to make the complex simple. After all, your end-users aren’t likely data scientists or AI experts, so it’s about enabling better decision-making based on advanced AI-powered analytics with ease of use, and in terms of consumption, offering a familiar dashboard experience.
Elevate your analytics with AI: download Unlocking end-to-end AI for analytics: From ML to GenAI.
What AI-powered dashboards mean for your end-users
AI dashboards empower end-users to make more informed decisions, save time and effort in formulating how and where to act, and enable them to make sound data-driven decisions faster. And by embedding AI dashboards in your application, you can deliver these benefits as part of your end-users everyday experience, a game-changer for their decision-making, and for your application.
AI dashboards often add the following elements, providing a more insightful and engaging experience for end-users:
- Be more proactive using predictive analytics: Adding an AI-powered dashboard to your application that incorporates predictive modeling and machine learning algorithms to forecast future trends and outcomes, such as demand forecasts or projecting sales trends can help your application’s users anticipate potential risks and opportunities, and enable them to achieve more proactive decision-making.
- Gain real-time insights on opportunities and risks: AI algorithms within dashboards that are embedded in your app can be used to immediately and automatically identify patterns, trends, exceptions, and anomalies in data. This can make it easier for them to immediately identify key areas to act on, without having to go hunting for the data or letting the moment pass by.
- Engage more easily with conversational analytics: AI dashboards can leverage natural language processing capabilities to enable your end-users to interact with data, using conversational queries. This can simplify the process of data exploration and analysis, making it possible to broaden the adoption of your application and analytics experience you are providing by making it accessible to a wider range of users. For example, your end-users can use conversational analytics built on generative AI to ask business questions using natural language, and even gain narrative insights into the data.
- Act confidently using prescriptive analytics: Finally, adding AI dashboards in your app can also incorporate prescriptive analytics, which use data and computational algorithms to recommend actions to optimize or make improvements. This can enable your end-users to understand what has happened in the past, make predictions about what will happen in the future, and recommend the best course of action, like launching a new marketing campaign due to the seasonality benefit.
Three examples: AI use cases in sales and marketing, finance and retail
Now that you know the components of an AI dashboard, let’s explore how you’d use them if you’re building one that includes some or all of the AI elements.
An AI dashboard for sales and marketing
Let’s say you make software for marketing teams to manage their social media campaigns. Your AI dashboard could leverage AI and ML algorithms to analyze social media data from Facebook, Twitter, Instagram, and LinkedIn. Your dashboard would feature traditional descriptive insights into the performance of social media campaigns, including metrics like engagement, reach, and conversion rates.
However, it could also provide predictive analytics to identify trends and patterns in audience behavior and enable marketers to understand what content resonates most with their target audience. Or you could give forecasts on future social media campaign performance trends, enabling marketers to anticipate and capitalize on upcoming opportunities.
To help marketers understand customer perception and adjust their strategies accordingly, you could use machine learning on the underlying data. The output would be advanced analytics that gauge the sentiment of social media conversations related to the brand or specific marketing campaigns.
With prescriptive analytics, you could add suggestions to your dashboard to give personalized content recommendations based on analyzing audience preferences and behavior. This would help your end-users tailor their content strategies more effectively.
An AI dashboard for finance and accounting
Consider that you make a finance application; one of the key audiences you want to engage is the Chief Financial Officer (CFO). If you could provide them with a powerful, actionable dashboard, they would become loyal users and be an important driver of customer retention.
Depending on the available data, we might give traditional descriptive analytics on financial metrics critical for CFOs. Metrics would include revenue, profitability, cash flow, liquidity ratios, and operational expenses. We’d show them year-over-year, period-over-period, to show how they change over time. Let’s be honest: While useful, that’s pretty much table-stakes, and not yet an AI dashboard.
But—let’s say we add some predictive analytics. The AI dashboard could generate financial forecasts and predictions using machine learning algorithms. It could analyze historical data, market trends, and other relevant factors to provide insights into future financial performance. This would help the CFO plan, budget, and identify potential risks and opportunities. Now we’re getting somewhere.
We could incorporate prescriptive analytics to enable cost optimization recommendations. For example, the dashboard could analyze cost structures and identify areas where cost optimization is possible. It would use machine learning to find patterns, trends, or anomalies in cost data and give recommendations to reduce expenses without negatively impacting business operations. This would help the CFO manage costs effectively and improve profitability, which would be a huge win.
Given our CFO may not be an advanced analytics user, we might also consider adding a conversational analytics component. That way, they could ask questions using natural language rather than requiring clicks and navigation, or use generative AI to get explanations behind some of the visualizations they are seeing.
An AI dashboard for retail
Here’s a final example for retail: Put yourself in the shoes of a product manager providing a dashboard for retail customers. We’re probably already providing retail performance metrics like revenue, sales trends, top-selling products, and customer purchase patterns. These classic descriptive analytics help retail managers identify opportunities for revenue growth and optimize product offerings. One way to enhance even core descriptive analytics here might be to offer stronger visualizations or weave insights into the core UX of our application.
But let’s take our retail dashboard further and power it up with AI. We could combine our historical sales data and market trends with external factors (weather or seasonality) to predict future demand for products or services. This would help our retail managers make data-driven decisions around inventory planning, production, and supply chain optimization, making the business much more capital-efficient.
For sentiment analysis, we might incorporate natural language processing techniques to analyze customer feedback from various sources, such as surveys, social media, or customer support interactions. This would help the retail manager understand customer satisfaction levels and forecast problematic satisfaction trends. This feedback analysis would be a powerful way for the retail manager to enhance the customer experience.
Read more: 5 Ways analytics is transforming retail and e-commerce.
Critical considerations for design and development
By now, you should have a real sense of the opportunity to shift from traditional dashboards to AI dashboards. If you’re a product manager or a developer, this is a powerful way to drive competitive advantage, engage your users, and excite your sales team. But what are some critical considerations to get started?
Use a composable SDK
The first consideration: Use a composable SDK built on a platform that provides various traditional and AI-based analytic services. A composable SDK is important for building an AI dashboard because it allows developers to integrate pre-built components into their applications easily. By providing a set of reusable and flexible building blocks, a composable SDK enables developers to quickly assemble and customize AI capabilities such as analytics based on machine learning models, natural language processing, and data visualizations within their dashboard interface
One example is Sisense’s approach, which offers Compose SDK with an Analytics Chatbot (beta)—a customizable and embeddable chatbot experience powered by LLM (Language Learning Models). This component is provided as a React Component and follows a composable architecture. Each aspect, such as QuickStart questions, Narratives, and Data Topics, is a discrete React Component, allowing developers to easily build and customize their AI-enabled chatbot within their dashboard or application. Because Sisense’s functionality can be accessed through an API, developers have complete control over integrating GenAI-powered analytics deeply into their applications.
This composable approach empowers developers to swiftly incorporate advanced AI capabilities into their AI dashboards while maintaining flexibility and control over the development process.
Use a robust analytics platform
The second consideration: Using an analytics platform that provides a full array of descriptive, predictive, and natural language capabilities, and the API-level flexibility to link prescriptive recommendations with their broader app, going from insight to action.
For example, Sisense has Sisense Forecast, which enables you to confidently forecast future values and achieve advanced forecasting capabilities using menu-driven, point-and-click capabilities. This way, you can immediately discern trends and patterns in data. With Trends, you can add another layer to visualizations to highlight the data trends and make them easier to consume. You can easily draw trend lines using several statistical algorithms, including exponential smoothing and local estimates. Compare trends with previous or parallel periods.
Meanwhile, Sisense Explanations automatically identifies the most probable key drivers for a given change in the data. It covers hundreds of possible contributing factors and presents the top ten fields (or combinations of fields) that contribute most significantly to the change in behavior.
With Sisense Pulse, users can continuously monitor KPIs, and when statistical outliers are detected, it alerts the user automatically.
As you can see, if you leverage an analytics platform, you can use pre-built functionality to get your AI dashboards to market faster.
Next step: Blaze your path to your first AI dashboard
You just learned about the power of AI dashboards, with examples that stretch across sales and marketing, finance and accounting, and retail, showcasing the potential for advanced analytics and better decision-making.
Even better, your AI dashboard future needn’t be that far away, as you can get started today. To learn more about analytics solutions that will keep you competitive, schedule a demo.
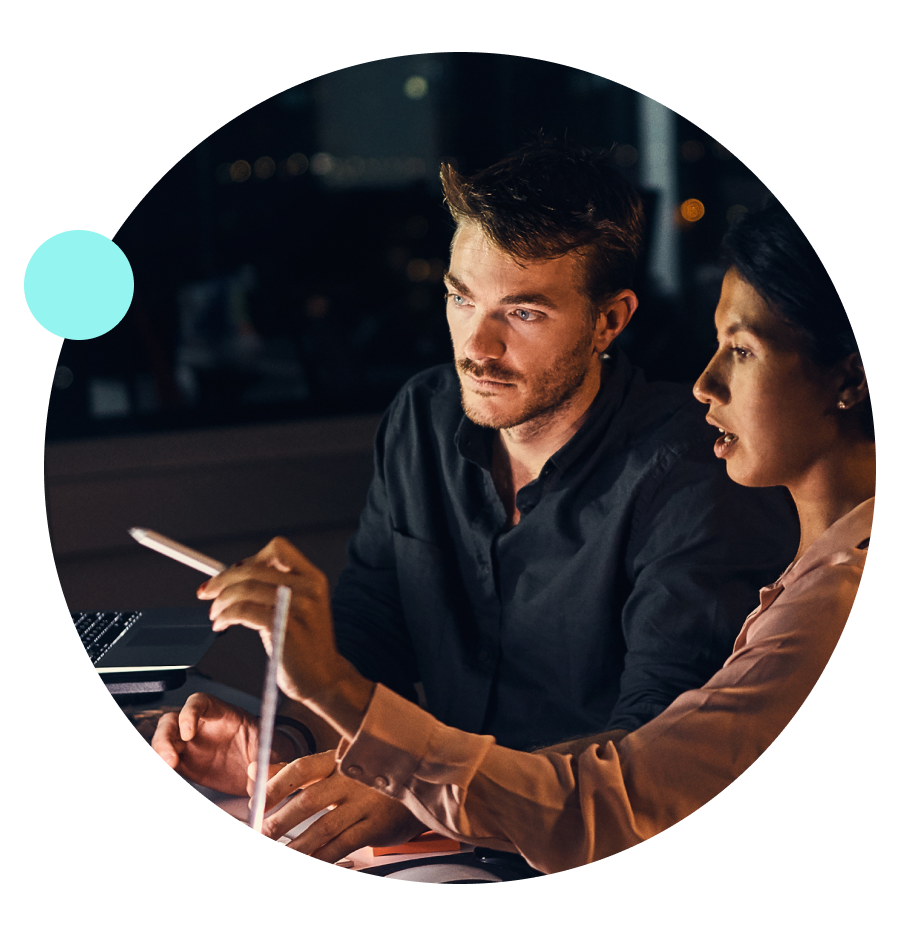