Harnessing AI predictive analytics for business transformation
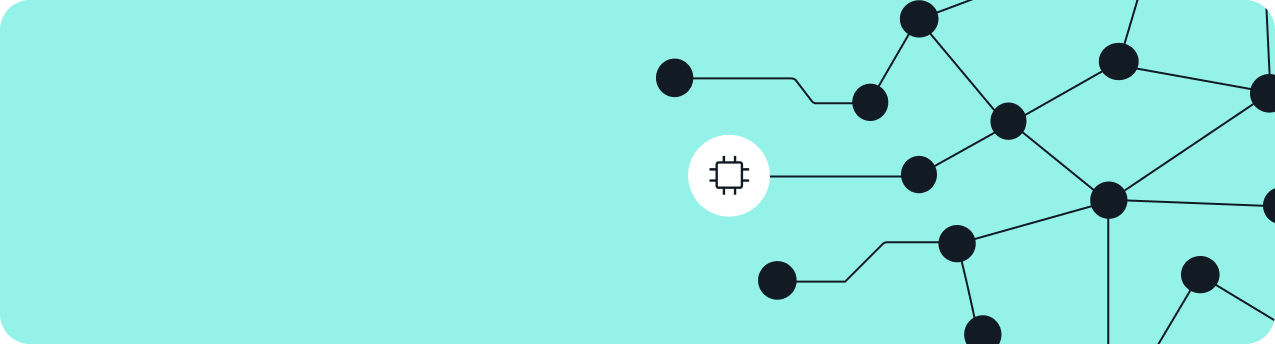
- Blog
- Business Perspectives
Artificial Intelligence (AI) in predictive analytics is revolutionizing the business world, offering unprecedented insights and competitive advantages. This article explores the transformative impact of AI on predictive analytics and its practical applications across various business sectors. What is AI predictive…
Artificial Intelligence (AI) in predictive analytics is revolutionizing the business world, offering unprecedented insights and competitive advantages. This article explores the transformative impact of AI on predictive analytics and its practical applications across various business sectors.
What is AI predictive analytics?
AI predictive analytics involves using artificial intelligence to analyze existing and historical data to predict future trends, behaviors, and outcomes. It leverages AI algorithms and machine learning to process vast datasets, uncovering patterns and correlations not easily identified through traditional analysis. Integrating AI enhances the power of predictive analytics, providing more precise, efficient, and dynamic forecasting.
AI predictive models: From theory to practice
It’s vital to understand AI’s role in predictive analytics. AI encompasses more than robotics; it’s about machines autonomously performing tasks. Machine learning, a core element of AI, discerns patterns in data, offering actionable insights.
Applications of AI in predictive analytics
AI’s use in predictive analytics is diverse, covering various industries. It predicts consumer buying trends, optimizes inventory in retail, forecasts market fluctuations, and aids investment decisions in finance, and in healthcare, AI anticipates patient outcomes, enhancing treatment approaches. AI’s adaptability makes it applicable in any field where predicting future events or behaviors is strategically beneficial.
AI predictive analytics methods can be applied to almost any business domain you care to think of. For example, repairing factory machines before they break, detecting fraud attempts, and keeping IT environments secure. Below, we’ve chosen three areas to look at more closely – sales, human resources, and supply chain analysis.
Case study: AI predictive models in sales
Consider AI predictive models analyzing sales data. Machine learning could identify purchasing patterns, like common product combinations that lead to repeat purchases. This knowledge enables targeted marketing, refining sales strategies through predictive AI.
AI predictive analytics across industries
AI predictive analytics is beneficial in areas like manufacturing, fraud detection, and IT security, demonstrating its wide-ranging adaptability.
Revolutionizing sales and retail
Predictive sales analytics, a mainstay for online retailers, are now essential across various sales models. AI plays a crucial role in resource allocation and customer experience enhancement, even in brick-and-mortar stores.
Although online sales companies may have pioneered the use of predictive sales analytics, other sales organizations are now getting in on the act. For instance, key account managers traveling to see customers on their premises for big-ticket items like aircraft or warehouse automation only have a small fraction of their total work time available for real selling. Predictive analytics can show them how best to allocate that selling time, using another kind of recommendation engine that says, “This other customer might also like what you already successfully sold elsewhere.”
Even brick-and-mortar retail outlets are using predictive analytics to boost sales. Coffee-house chain leader Starbucks has already announced it will deploy an AI-based recommendation system in its establishments worldwide.
A quick question for you—how much do you think you could improve your business by using recommendation engines or similar predictive sales analytics? Research firm Aberdeen found that companies homing in on customer needs and wants through predictive analytics increased their organic revenue by 21% year-on-year, compared to an industry average of 12%.
Enhancing supply chains
Supply chain optimization is another key application of AI predictive analytics. To keep customers satisfied while staying profitable, supply chains must ensure the right products are made in the right quantities and then stored in the right places, such as retailers’ shelves, distribution centers, and manufacturers’ depots.
AI predictive analytics for retail can help your supply chain perform better by showing where demand is likely to be strongest, and which retailers and distribution centers will need which kind of replenishment. It can also suggest which transport conditions (weather, congestion, even industrial action) will affect the optimal choice of transport modes and routes. With the right interface to your analytics, you could also get up-to-the-minute forecasts of production and shipment requirements simply by asking for them out loud to your system.
Pharmaceutical companies, for example, can use AI predictive analytics to refine drug sales and supply forecasts in the light of events like hospital fires or other disasters. Supermarket chains can factor in local weather patterns into analytics to predict location by location which product shipments to make. For example, more sun lotion and barbecue sets for a sunny, summer weekend in one place, more cans of soup for rainy weather in another!
Transforming human resources
Human resource departments are aware of the cost of employee churn to their enterprise. High turnover leads to high costs to recruit replacement workers and lead time to train new employees before they can become productive.
In companies where this is a significant problem, predictive analytics can come to your aid, perhaps even coming up with some surprising insights. Using data from different sources, you may find that previous tactics such as higher pay, promotions, and higher performance ratings did not reduce churn. Instead, in various combinations, such as receiving a promotion without a corresponding pay raise, they increased it.
Other companies use predictive HR analytics to predict which managers will do well and which could use additional management training to head off personnel catastrophes before they happen. As a cautionary note, however, companies also make a point of using these analytics to help their HR teams make decisions, but not to take over the decision-making process. Predictive analytics in HR, as in other areas, often have the most to offer when used together with human experience and judgment.
Starting your AI predictive analytics journey
Your AI predictive analytics can only be as good as the data you feed them… And the ability of your analytics platform to cope with the necessary volume and diversity of data.
Further statistics from Aberdeen show that companies achieving the best results with predictive analytics tend to use multiple data sources. They include unstructured, text-based, machine, or Internet of Things (IoT) data. The more data you can analyze and the faster you can analyze it also means the more likely you are to get viable, actionable insights in a timely way.
It’s important to look for a platform that allows you to easily bring in, clean, and mash up all kinds of large datasets for results like those described here. After all, you didn’t think we were going to tell you how great predictive analytics could be for your business without giving you a good solution for getting them, did you?
To learn more about AI-driven analytics, check out our latest guide, Unlocking end-to-end AI for analytics: From ML to GenAI.
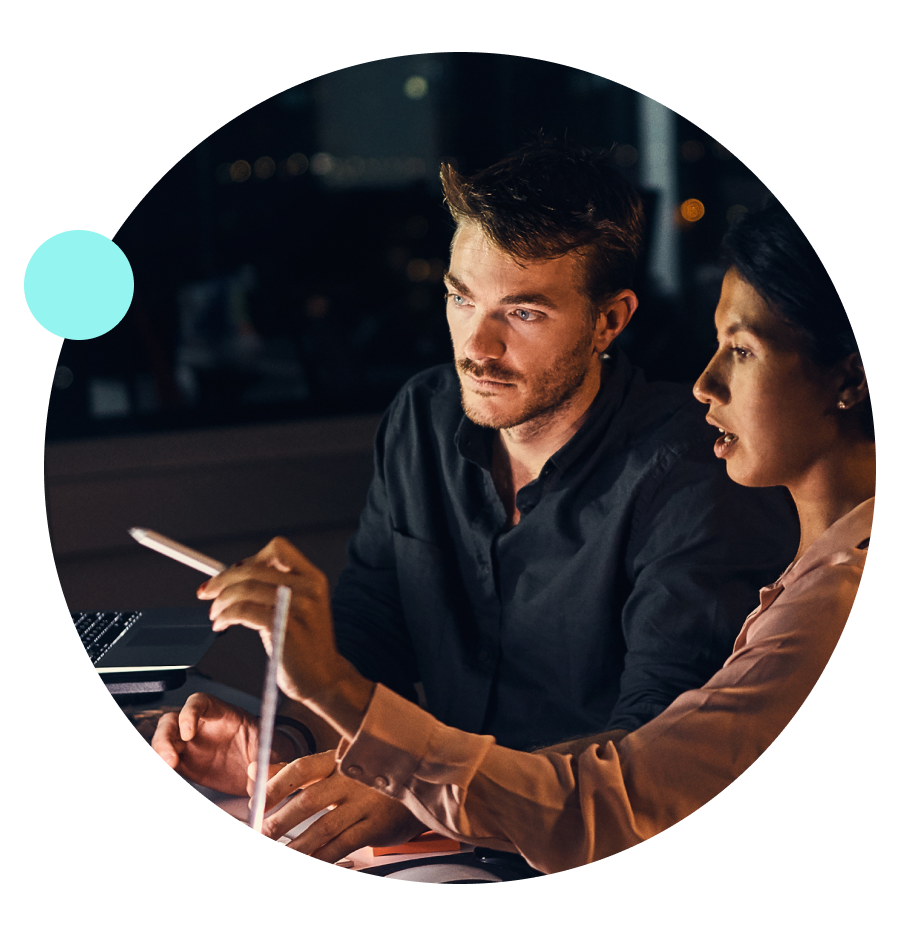